Progetti
Progetto Probabilistic Space-time models for forest fire spreading
Progetto Mathematical modelling of fire propagation in forests
Articoli
Modelling brain dynamics by Boolean networks
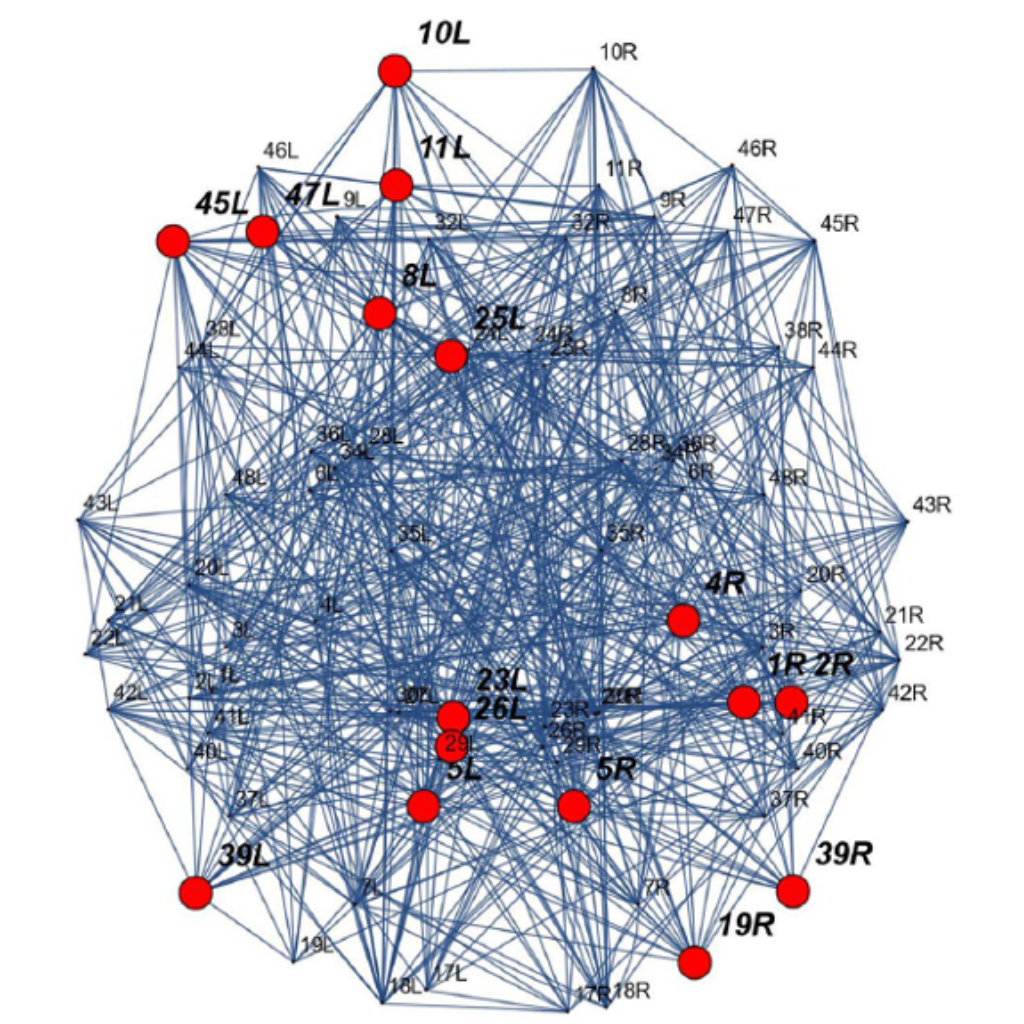
Scientific Reports
Francesca Bertacchini, Carmelo Scuro, Pietro Pantano, Eleonora Bilotta
Understanding the relationship between brain architecture and brain function is a central issue in neuroscience. We modeled realistic spatio-temporal patterns of brain activity on a human connectome with a Boolean networks model with the aim of computationally replicating certain cognitive functions as they emerge from the standardization of many fMRI studies, identified as patterns of human brain activity. Results from the analysis of simulation data, carried out for different parameters and initial conditions identified many possible paths in the space of parameters of these network models, with normal (ordered asymptotically constant patterns), chaotic (oscillating or disordered) but also highly organized configurations, with countless spatial–temporal patterns.
SARS-CoV-2 emerging complexity and global dynamics
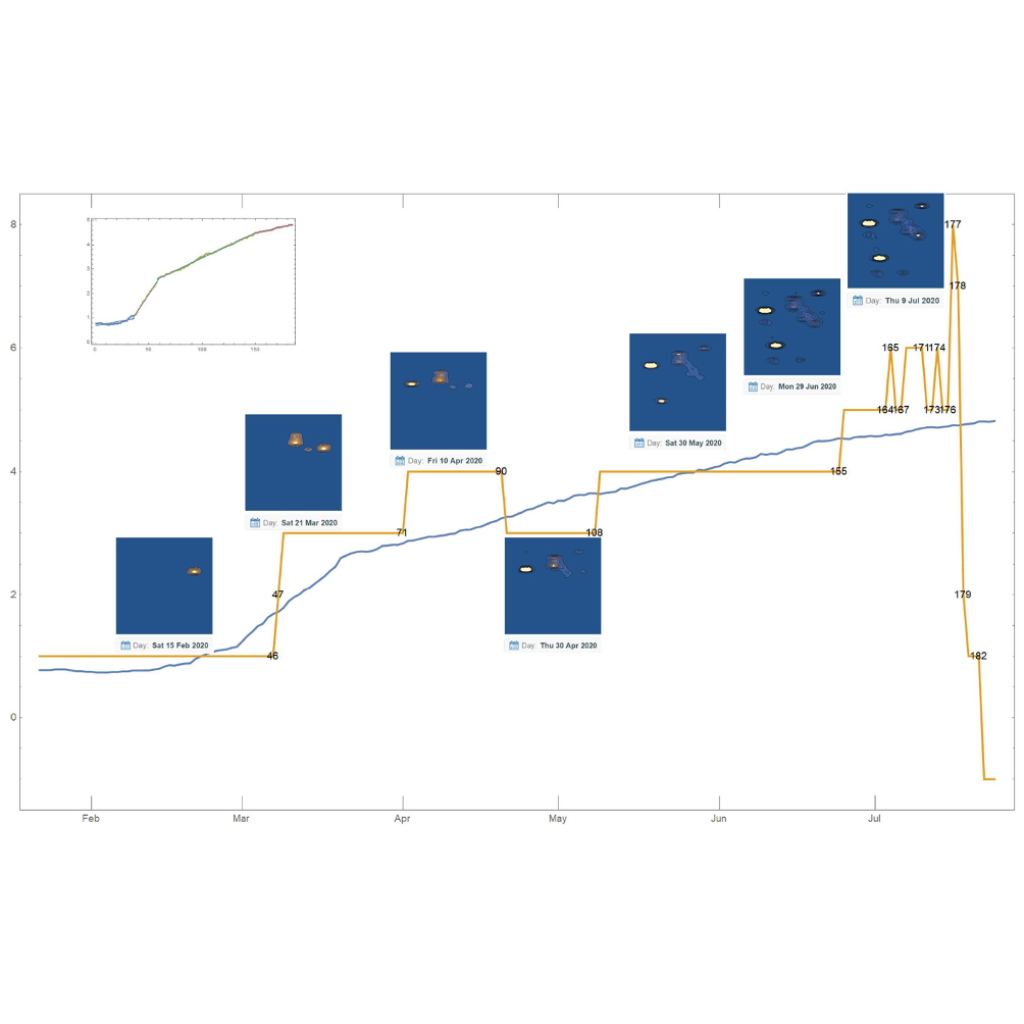
Chaos: An Interdisciplinary Journal of Nonlinear Science
Francesca Bertacchini, Pietro S Pantano, Eleonora Bilotta
The novel SARS-CoV-2 virus, prone to variation when interacting with spatially extended ecosystems and within hosts, can be considered a complex dynamic system. Therefore, it behaves creating several space–time manifestations of its dynamics. However, these physical manifestations in nature have not yet been fully disclosed or understood. Here we show 4D and 2D space–time patterns of the rate of infected individuals on a global scale, giving quantitative measures of transitions between different dynamical behaviors. By slicing the spatiotemporal patterns, we found manifestations of the virus behavior, such as cluster formation and bifurcation. Furthermore, by analyzing morphogenesis processes by entropy, we have been able to detect the virus phase transitions, typical of adaptive biological systems.
Enhancing store layout decision with agent-based simulations of consumers’ density
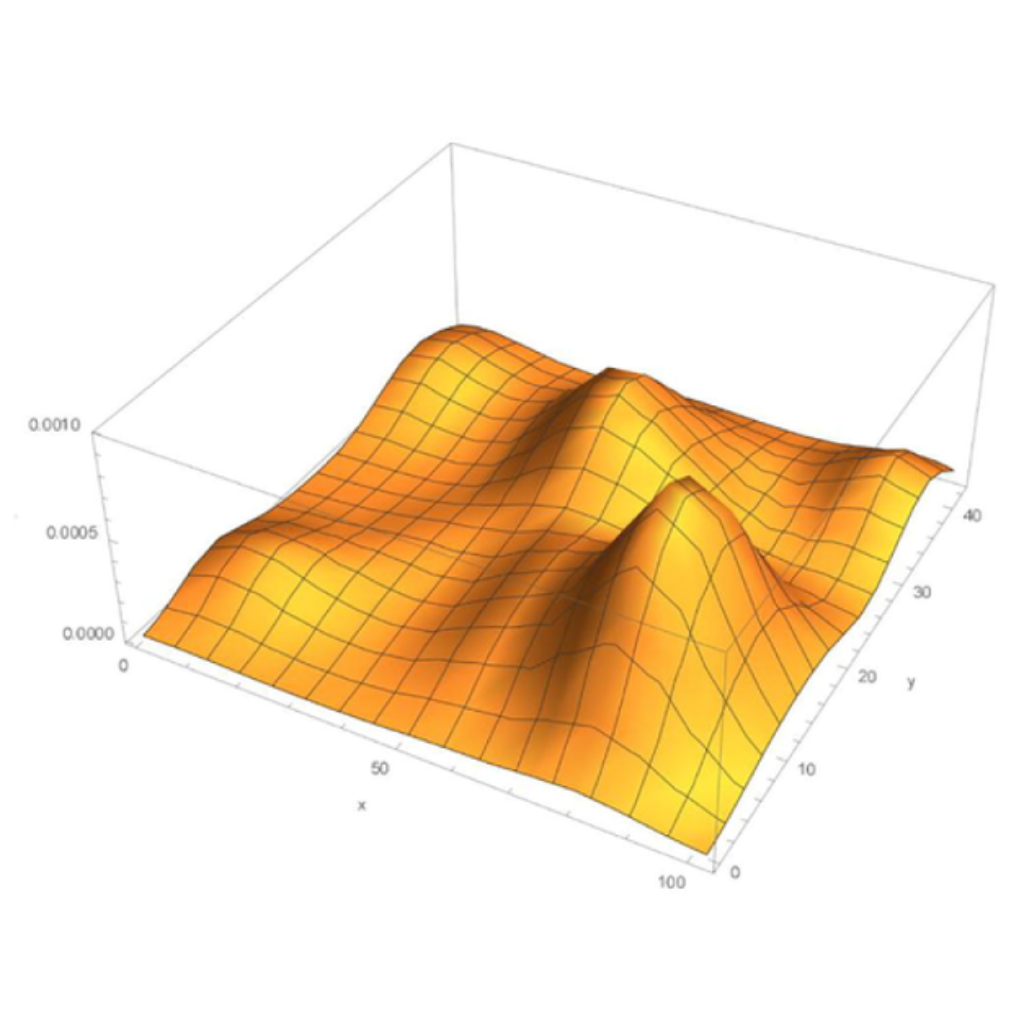
EXPERT SYSTEMS WITH APPLICATIONS
Eleonora Pantano, Gabriele Pizzi, Eleonora Bilotta, Pietro Pantano
Customer concentration inside a store is of pivotal importance for retail management, acquiring controversial contributions about the best number of consumers in the floor space to ensure an enjoyable and pleasant experience. Indeed, the excessive concentration of people (crowd) might discourage from shopping in that location, while on the other hand, a certain traffic to the store generates profit for retailers.The aim of this paper is to support retailers’ informed decisions by refining our understanding of the extent to which store layouts influences consumer density. To this end, we conduct a large field study using a unique dataset covering customers in a real grocery store with agent-based simulations. Results clearly show the extent to which this kind of simulations help predicting the changes in store layout able to affect customer density in the areas, while ensuring the same number of individuals.
A unifying nonlinear probabilistic epidemic model in space and time
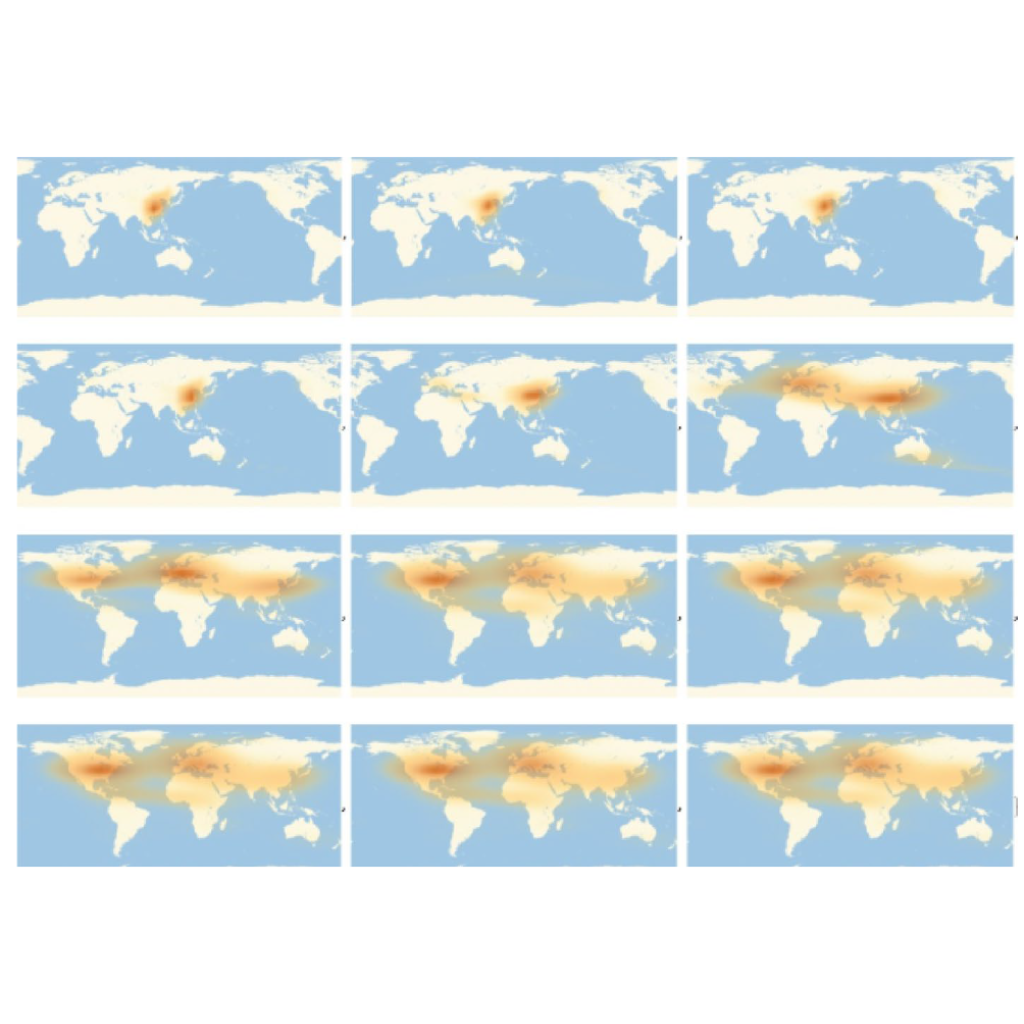
Scientific Reports
Roberto Beneduci, Eleonora Bilotta, Pietro Pantano
Covid-19 epidemic dramatically relaunched the importance of mathematical modelling in supporting governments decisions to slow down the disease propagation. On the other hand, it remains a challenging task for mathematical modelling. The interplay between different models could be a key element in the modelling strategies. Here we propose a continuous space-time non-linear probabilistic model from which we can derive many of the existing models both deterministic and stochastic as for example SI, SIR, SIR stochastic, continuous-time stochastic models, discrete stochastic models, Fisher–Kolmogorov model. A partial analogy with the statistical interpretation of quantum mechanics provides an interpretation of the model.
Multi-step prediction method for robust object tracking
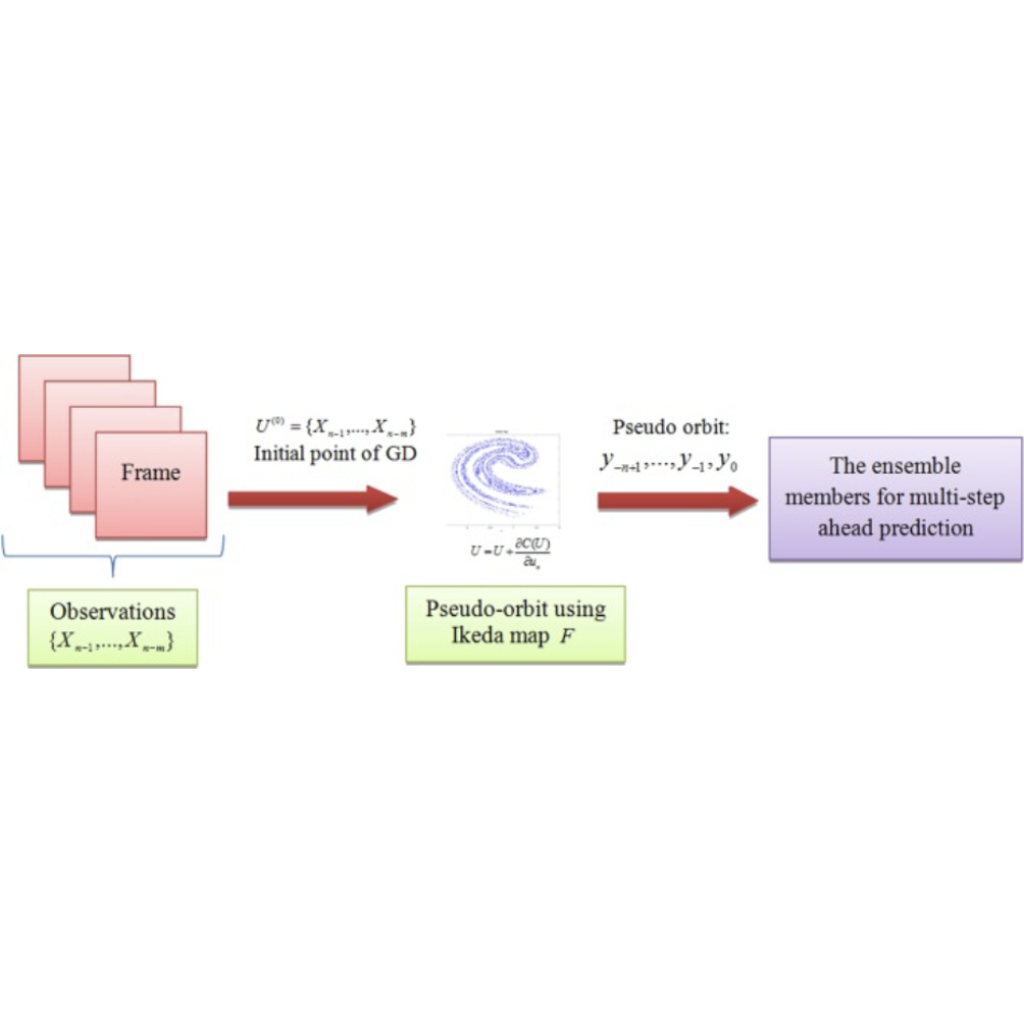
DIGITAL SIGNAL PROCESSING
Marjan Firouznia, Karim Faez, Hamidreza Amindavar, Javad Alikhani Koupaei, Pietro Pantano, Eleonora Bilotta
In this paper, multi-step ahead prediction method for object tracking based on chaos theory is introduced. The chaos theory is used to preserve the information of object’s movement and to model uncertainty and nonlinearity of movement in video sequences. The methodology of the algorithm includes three steps. First, adaptive pseudo-orbit data assimilation is applied to estimate the next state by using the previous states of object. Second, the ensemble members of the state are generated to predict multi-step prediction. Then, the likelihood function of members selects candidate patch for target detection using color information. The algorithm significantly reduces the prediction error because of high-order dynamical information of motion and chaotic prediction. To verify the efficiency of the tracker, the tracking algorithm is compared with the stochastic and deterministic methods under two datasets.
Chaotic target representation for robust object tracking
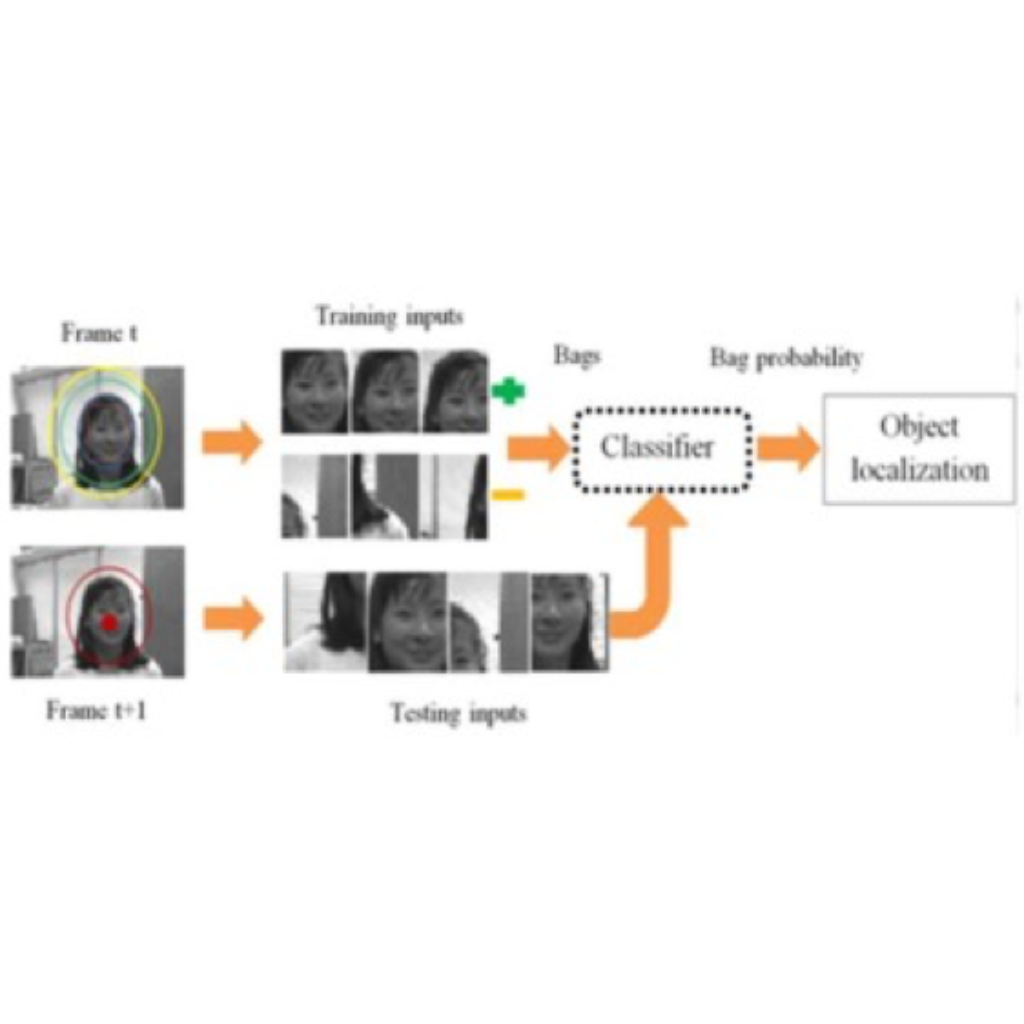
SIGNAL PROCESSING: IMAGE COMMUNICATION
Marjan Abdechiri, Karim Faez, Hamidreza Amindavar, Eleonora Bilotta
In this paper, a new object representation method is introduced as an appearance model based on chaos theory. For robust object tracking, the theory is used to extract a deterministic model from irregular patterns of pixel amplitudes in a target region. The object tracking algorithm that accompanies the proposed method involves two steps. First, fractal theory is applied to a compressive sensing method intended to embed an image into a two-dimensional state space during tracking by detection. After an object representation is extracted from an instance, the fractal dimension of the state space is assigned to the importance weight of the instance for efficient online multiple-instance learning. Second, a chaotic map approach is adopted to update the appearance model. Such updating is a crucial step in selecting discriminative and robust features.
The chaotic dynamics of high-dimensional systems
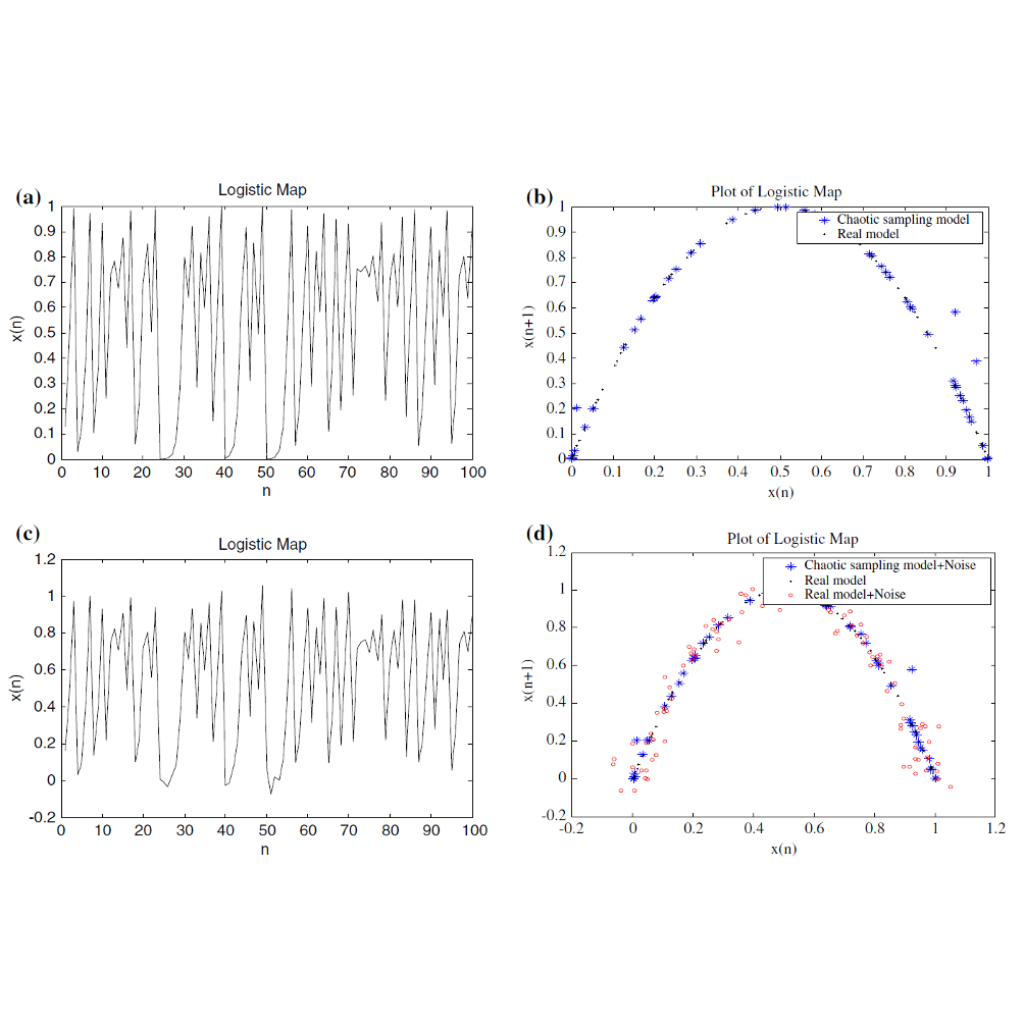
NONLINEAR DYNAMICS
Marjan Abdechiri, Karim Faez, Hamidreza Amindavar, Eleonora Bilotta
This paper introduced a new method to exploit chaotic, sparse representations of nonlinear time series data. The methodology of the algorithm included two steps. First, the proposed method applied the fractal theory to estimate optimal dimension in a compressive sensing of a time series, and sparse data were concentrated on a pseudo-orbit trajectory. Second, the chaotic trajectory was extracted from the data obtained from the first step by employing a chaos prediction method. To verify the efficiency of the proposed method, the algorithm is applied to three categories, consisting of chaotic noise reduction, signal compression, and image compression. The experimental results indicated that the proposed method outperformed other state-of-the-art methods with up to a 95% reduction in errors.
Speeding up cellular neural network processing ability by embodying memristors
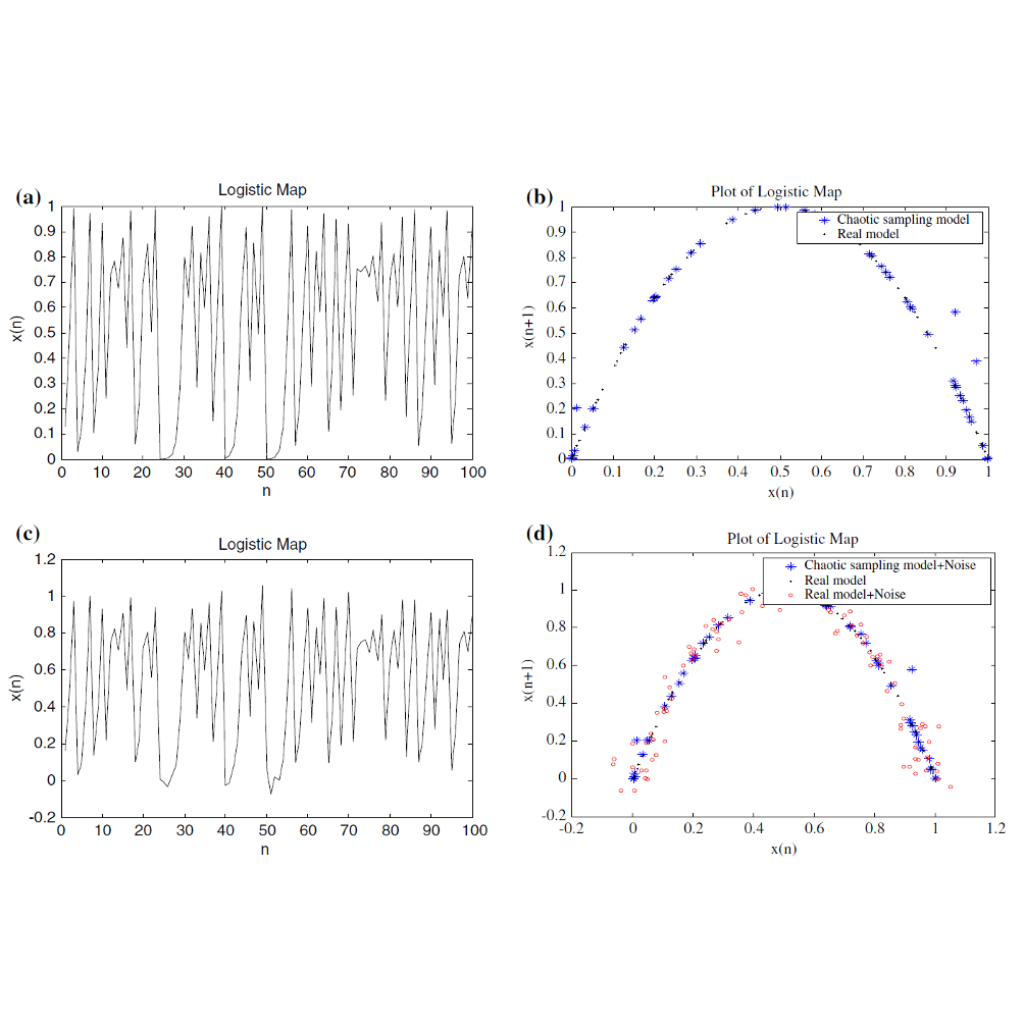
IEEE TRANSACTIONS ON NEURAL NETWORKS AND LEARNING SYSTEMS
Eleonora Bilotta, Pietro Pantano, Stefano Vena
Cellular neural networks (CNNs) are an efficient tool for image analysis and pattern recognition. Based on elementary cells connected to neighboring units, they are easy to install in hardware, carrying out massively parallel processes. This brief presents a new model of CNN with memory devices, which enhances further CNN performance. By introducing a memristive element in basic cells, we carry out different experiments, allowing the analysis of the functions traditionally carried out by the standard CNN. Without modifying the templates considered by the scientific literature, this simple variation originates a significant improvement in ~ 30 % of performances in pattern recognition and image processing. These progresses were experimentally calculated on the time the system requires to reach a fixed point.
Complexity and emergence of wave dynamics in a chain of sequentially interconnected Chua circuits
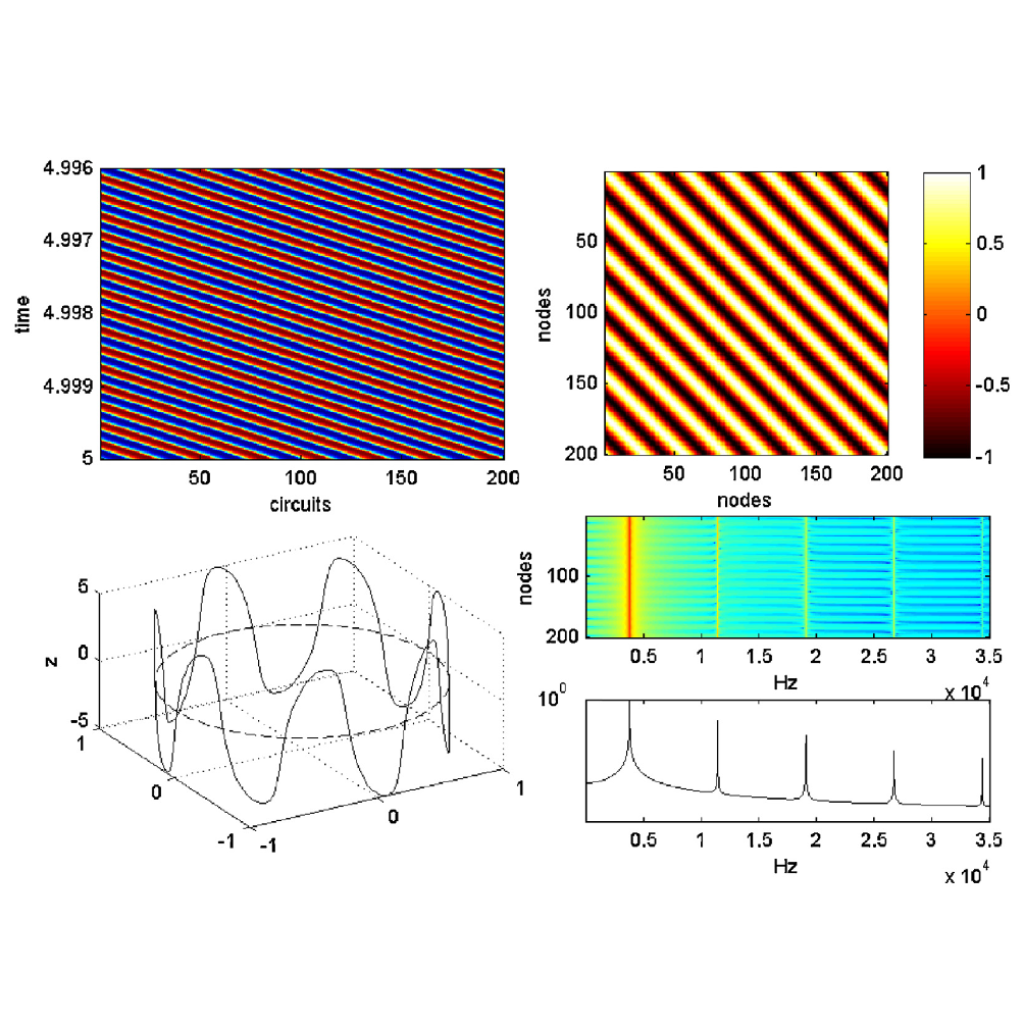
MECHANICS RESEARCH COMMUNICATIONS
Eleonora Bilotta, Francesco Chiaravalloti, Pietro Pantano
This paper considers an ensemble of Chua oscillators bidirectionally coupled in a ring geometry where locally coupled circuits form a closed loop of signal transmission. The spontaneous dynamics of this system is studied numerically for different coupling strength. A transition from periodic to chaotic regimes is observed when the coupling decreases. In the former situation, characterized by high coupling, all the circuits oscillate with pseudo-sinusoidal dynamics on periodic attractors; in the latter they evolve on the same-type of chaotic attractor with a progression of the dynamics from the Chua’s spiral to the double scroll as the coupling decreases. The emerging global dynamics is markedly different in the two cases and a phase transition between highly ordered and highly disordered global dynamics is observed.
Cellular nonlinear networks meet KdV equation: A new paradigm
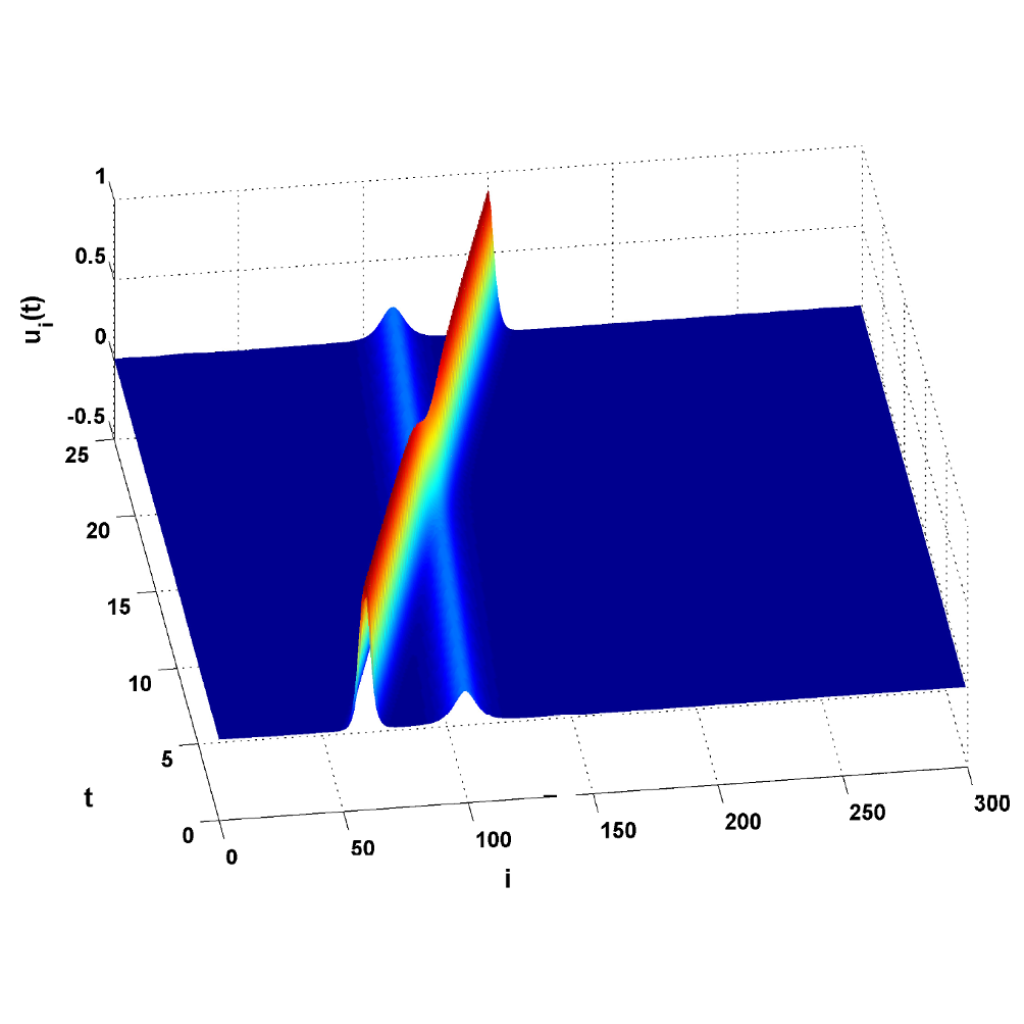
INTERNATIONAL JOURNAL OF BIFURCATION AND CHAOS
Eleonora Bilotta, Pietro Pantano
The Korteweg–de Vries equation, used to describe long and solitary wave propagation on shallow water, later called soliton, besides describing phenomena ranging from the dynamics of plasmas to tsunami can be implemented into Cellular Neural Networks (CNNs), analog circuit which processes signals in real time with the aim to create a completely innovative generation of transmission lines and a new method for studying wave-like propagation of information in multi-dimensional nonlinear and dispersive media. In this paper, after a short presentation of both systems, we empirically verify that the behavior of the KdV can be emulated by CNNs.
FPGA-based distributed computing microarchitecture for complex physical dynamics investigation
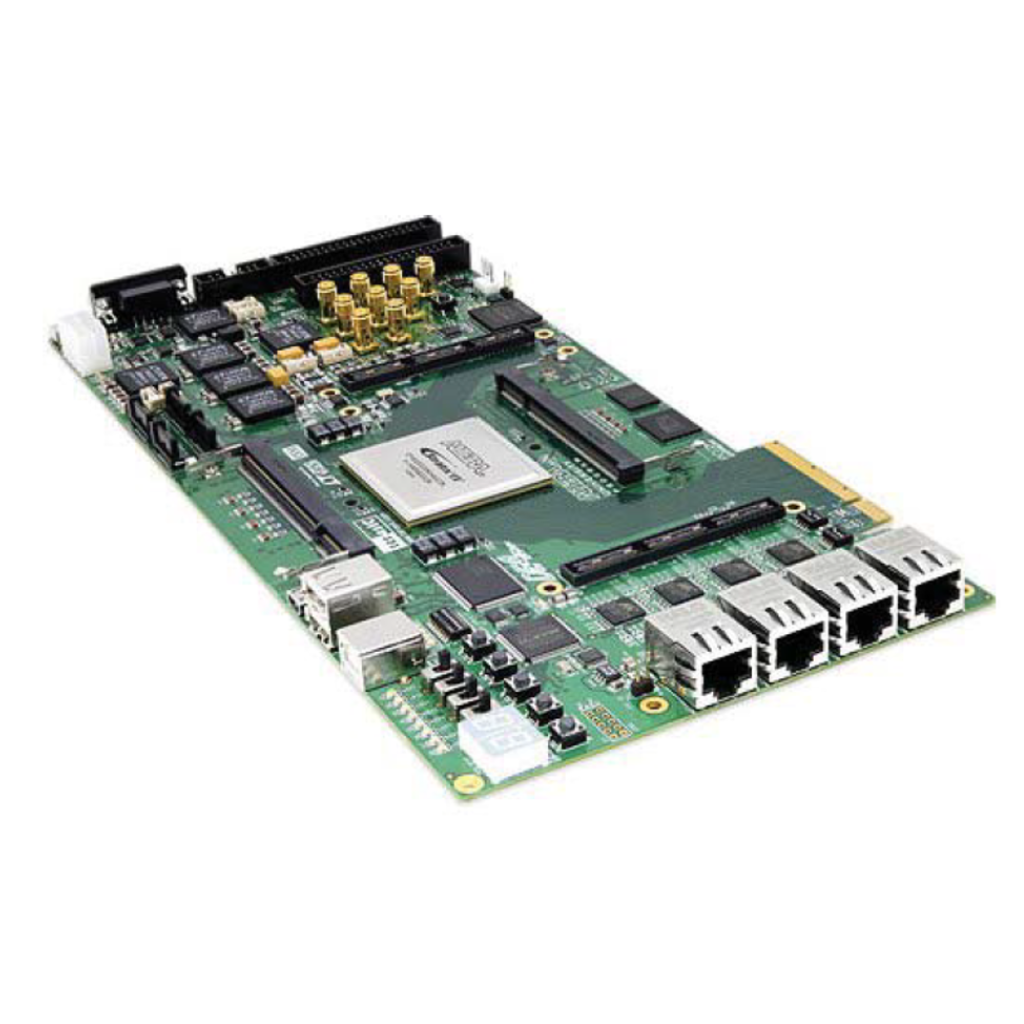
IEEE TRANSACTIONS ON NEURAL NETWORKS AND LEARNING SYSTEMS
Gianluca Borgese, Calogero Pace, Pietro Pantano, Eleonora Bilotta
In this paper, we present a distributed computing system, called DCMARK, aimed at solving partial differential equations at the basis of many investigation fields, such as solid state physics, nuclear physics, and plasma physics. This distributed architecture is based on the cellular neural network paradigm, which allows us to divide the differential equation system solving into many parallel integration operations to be executed by a custom multiprocessor system. We push the number of processors to the limit of one processor for each equation. In order to test the present idea, we choose to implement DCMARK on a single FPGA, designing the single processor in order to minimize its hardware requirements and to obtain a large number of easily interconnected processors.